Talks about data science are dominated by the latest machine learning algorithms, data engineering practices and cool applications of AI products. Ethics in data science however, is the most important and the least talked about topic. This is partly due to the topic being relatively "unsexy" but also due to it being a hard problem to solve.
As the AI industry matures, data professionals will need to abide by a strong code of ethics just like doctors today. In recent times, we are already seeing instances of commercially deployed AI algorithms showing proof of gender bias and racial bias. These are issues that we can attribute to training data smeared by our human flaws. But there are other ethical issues that can catch us off-guard such as the trolley problem in autonomous driving and the AI control problem with superintelligent AI agents.
Let's take a look at what can go wrong in data science and how ethics applies by taking a look at the following 3 stages of the data science cycle: data collection, ML algorithm training and AI decision making (inference). Then we will look into how ethics plays a crucial role.
DATA COLLECTION
To train any machine learning algorithm, a lot of data is needed. Depending on the application, the data source can vary. Netflix for example uses your movie & tv show rating data along with watch time and your search history to train a recommendation system in order to provide you with the best recommendations. Similarly the AI algorithm that powers the smart reply feature in Gmail learns from a huge array of emails to learn how to predict the user's next email reply.
In a lot of these cases, you would voluntarily opt in to provide the information for the AI algorithms, but too often that's not the case. This is true when using Google Search, which is constantly monitoring your search behaviour to serve you the best ads possible. In this case you don't have the option to stop seeing ads which might hinder your user experience. At the end of the day, you are the product when you sign up for these platforms for free and this keeps Google chugging along. If it's any consolation you can control what types of ads you see by going to https://adssettings.google.com/.
Solid efforts have been made by the European Union to shift the control of personal data back into the consumer's hand. The General Data Protection Regulation or GDPR, rolled out by the EU in 2016, explicitly sets out guidelines for private data use (user name, IP address, cookie data, etc). These are to be followed by any business that has a European entity or is dealing with any European citizens. This has been done in an effort to reduce the impact of data leakage and prosecute unlawful use of user data. Businesses are required to provide users with easier access to their personal data as well as retain the right to request their data to be omitted. Fortunately, the fruits of GDPR are already coming to fruition. Recently Whatsapp was fined US$267 million for failing to provide transparency around how they used user data.
While it's great seeing governmental institutions regulating the use of data by private companies, the onus is also on the data scientist to question sources of data, and work with their company to adhere to the highest degree of ethical practices. This can be difficult in the current landscape due to the fears of employment repercussions. However, if there existed a data science board similar to a medical board that regulated the industry, data professionals would be more empowered to act as whistle blowers.
TRAINING ML ALGORITHMS
During the model training step, the algorithms don't just learn to recognise patterns but they also learn the flaws of the training data. In 2014, Amazon created an AI algorithm to streamline their technical recruitment process after being fed 10 years worth of resume data. By 2015, the algorithm was found to be gender biased as it unfairly penalised women's resumes due to the data set being predominantly male. This famous tale is often used as a poster child for AI gone wrong. The AI simply learned what it was shown (garbage in garbage out) highlighting the need for an increased involvement of data scientists in policing the algorithm.
The complexity of many machine learning algorithms such as neural networks can lead to a roadblock when trying to detect bias and discrimination in the program. However, the PAIR team at Google is making some strides in this domain. They have released the What If Tool which allows you to plug in your AI models and interrogate various fairness metrics to evaluate the quality of your model outputs as well as your input data. The integration of similar systems in existing machine learning systems could significantly improve the overall quality of the pipeline as well as give stakeholders a higher degree of confidence in the fairness of the model output as it goes into production.
AI DECISION MAKING
Finally let's talk about what happens when you have a crucial system powered by AI algorithms. An autonomous car has a range of cameras and sensors that power an AI algorithm which makes driving decisions on the fly. The use of such a system is, for the most part, groundbreaking as it will end up saving thousands of lives, given that 90% of car accidents in NSW, Australia happen due to human error. However there is a huge dilemma encompassing self-driving cars, and this is the trolley problem.
Imagine that there's a train going full steam ahead down the track. At the end of the track, there are five people and now imagine you're at a lever which when pulled can deviate that train onto a second track where there's only one person. The question is whether you should allow the train to kill five people or one person. It's a complicated decision to make even for a human. How do you decide a person's worth? This is exactly the decision a self-driving car could be forced to make if it was on the road faced with deviating to the right or left to avoid the obstacle straight ahead.
How should the algorithm decide? This is most definitely not a technical question as the problem now extends outside of data science and engineering. Luckily cutting edge companies like DeepMind are recognising this fact and appointing an independent ethics and society board to oversee the impact of its technology on society. I'd argue that this is a step that needs to be taken by most companies regularly using AI models.
There is no denying that AI has come very far in the past 2 decades from a technological perspective. But, as we look into the future of AI, there is a pressing need to empower data scientists to apply more rigid ethical frameworks in their daily tasks.
Written by Intelligen consultant & Data Scientist,
Samanvay Karambe
Tag :- Data Science, AI, Artificial Intelligence, Machine Learning
These Posts are Suggested For You
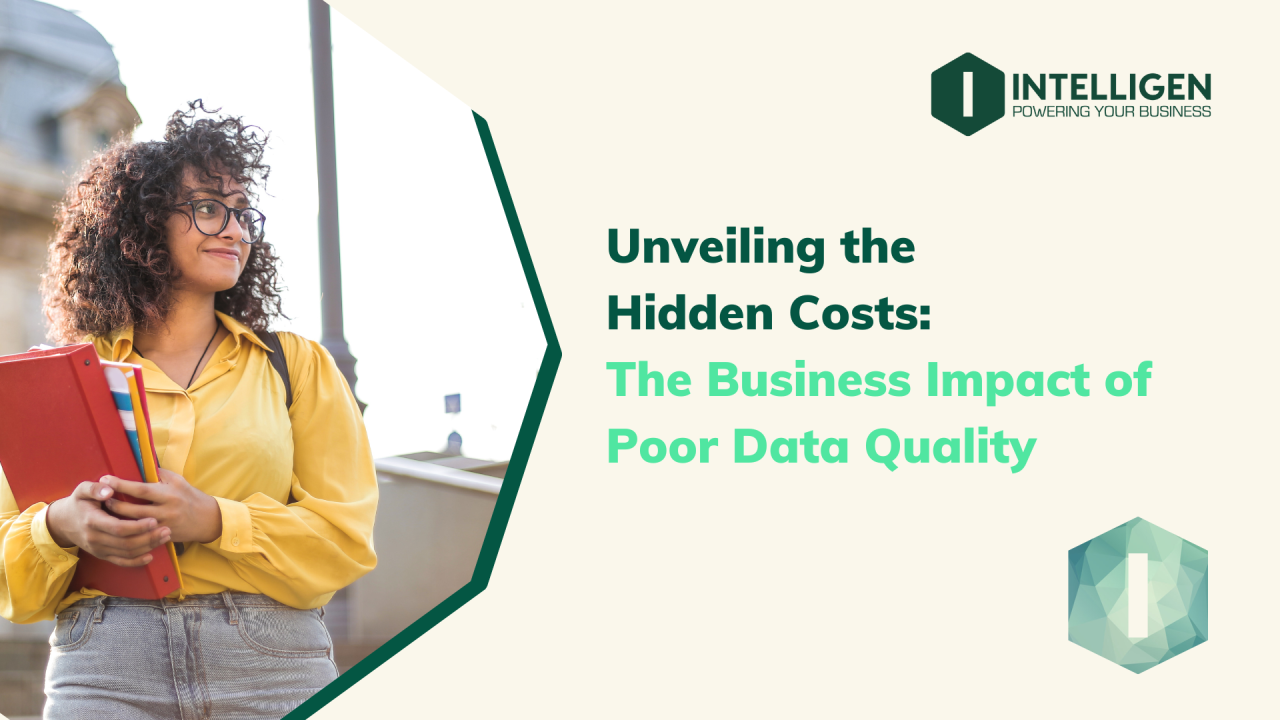