It is becoming increasingly evident that the effectiveness of AI systems is intricately linked to the quality of the data they utilise. Data accuracy, consistency and reliability serves as bedrocks for any efficient AI system.
Confronting Data Quality Challenges
- Data Accuracy: The accuracy of data is central to the performance of AI systems, even minor inaccuracies can have a substantial negative impact.
- Data Consistency: Consistency in data is equally crucial, inconsistencies can result in inaccurate risk assessments. Reports from regulatory bodies have revealed that inconsistencies in financial data among major banks can lead to a substantial 20% variance in risk modeling outcomes.
- Data Quality: Within the financial sector, regulatory bodies like the Australian Prudential Regulation Authority (APRA) emphasize the critical importance of data quality. Poor data management can lead to misinformed decisions, customer complaints, and regulatory actions, impacting operational efficiency and reputation.
- Data Capture: accurately capturing relevant, necessary information poses significant challenges, both in terms of identifying and ascertaining the data.
Strategic Approaches to Data Management
Addressing these challenges requires strategic data management approaches:
- Regular audits of data and cleaning processes are essential for ensuring data accuracy and reliability.
- Training AI systems with 'dirty data' can enhance their resilience to real-world imperfections.
- Data annotation and labeling are critical for supervised learning models.
Professional Recommendations with Industry Context
- Investing in high-quality data sources is crucial for sectors like energy, where predictive maintenance relies heavily on data accuracy. Using top-quality data sources can lead to a reduction in downtime by up to 25%, significantly impacting efficiency and safety.
- Enhancing data literacy among employees serves as a frontline defense against fraudulent transactions.
- Cloud-based data cleaning tools offer scalability and flexibility, essential for handling large data volumes in sectors like e-commerce.
- Collaborating with data cleaning specialists ensures adherence to best practices and maintainable standards, reducing operational risks.
- Adopting a continuous improvement approach to data cleaning is essential, where market conditions necessitate
agility in data management.
Conclusion
In conclusion, ensuring high-quality data for AI systems is a challenging yet manageable task with the right strategies in place. As AI continues to evolve, the importance of data quality will only grow, promising to revolutionize industries and drive business success.
Discovering the Linkage: Get started in understanding the connection between the quality of your data and your success in AI maneuver.
Reach out, and let's link your AI success story.
Subscribe to our newsletter for more valuable insights:
info@intelligengroup.com
02 9188 3911
Featuring SARA AI by Intelligen! For more information, visit this link: https://drive.google.com/file/d/10g1m3RFKFQ9P9iVfRQftaFz4NqwymCyo/view
These Posts are Suggested For You
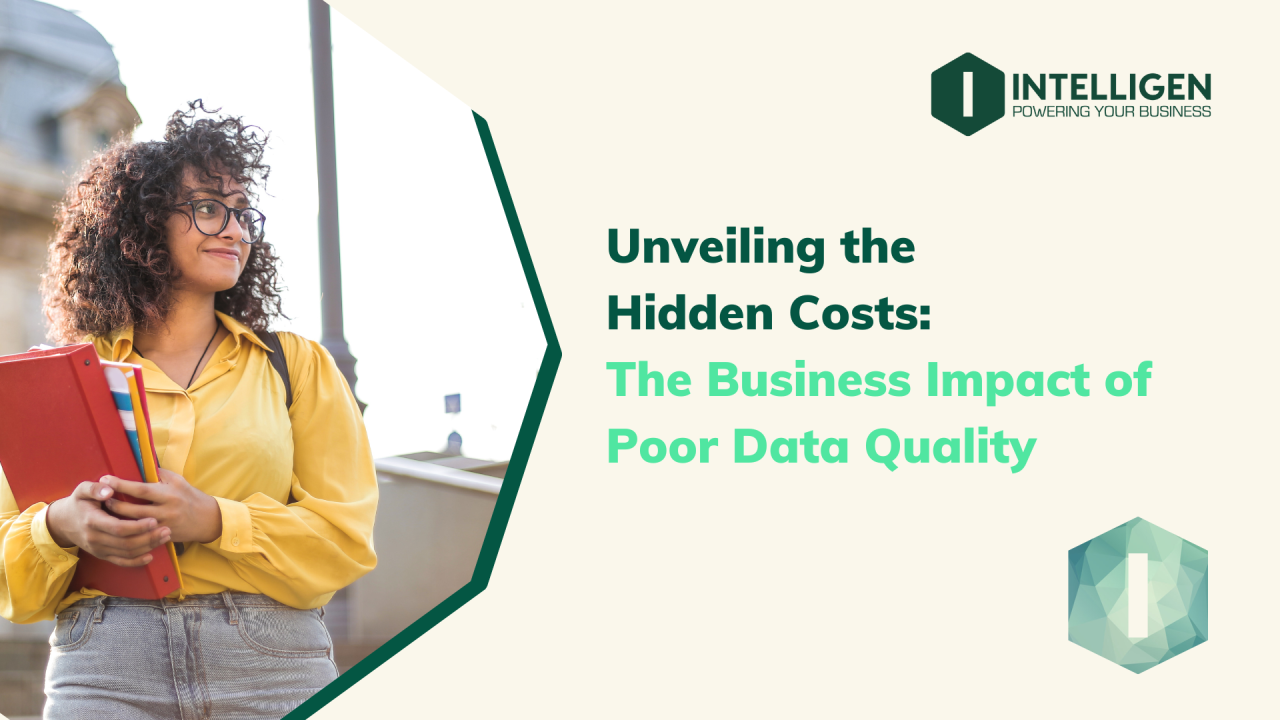
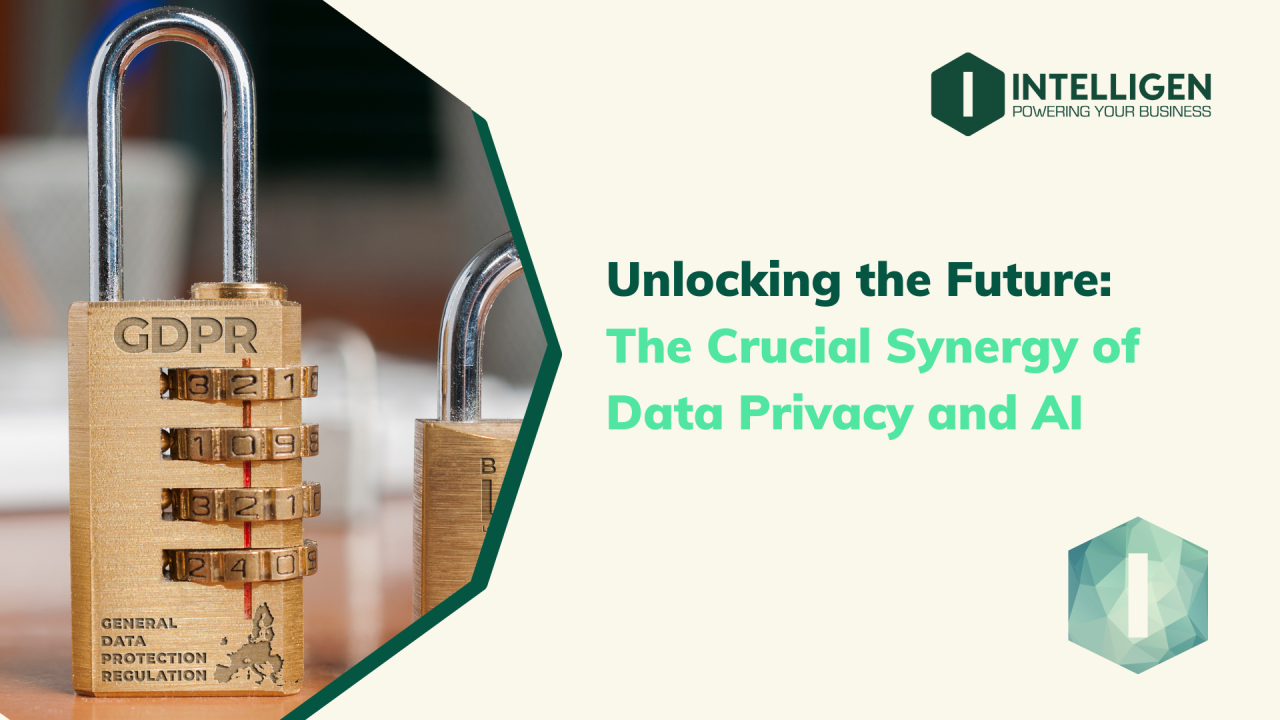